Наши популярные онлайн курсы
Every time risk professionals would have a conversation about risk analysis and specifically risk quantification, someone, inevitably, would come in on a high horse and use this excuse to supposedly undermine the conversation. The argument is completely false and yet, sure as day and night, someone always repeats it.
See if you can spot it in the quotes below from the comments to one of my posts today:
If you venture into quantifying risk of loss, you best be close in your accuracy, otherwise the audience will have the means to measure your inaccuracy and therefore their faith in your overall program.
So you will use your method to predict your cybersecurity attacks and losses for the next 6 months and publish it (internally) for an audit review for accuracy?
Alex has created a short bootcamp designed to help companies implement quantitative risk management. Imagine saving the company so much money that investing in risk management competencies and resources becomes a no brainer for the executives. That’s exactly what Alex Sidorenko did at a global $10B chemical company and he has been kind enough to share his top tips and lessons learned with you each week. Sign up now!
Shock events typically disrupt. Historical distributions and correlations suddenly shift. Those events can destroy many or enrich the lucky. The math hits a wall.
No distribution accurately predicts if China will attack Taiwan and when. And yet it’s a genuine security risk. Nor managed to predict when Russia attacked Ukraine.
Have you come across similar arguments before? The lame excuse usually goes something like this – risk modelling is not perfect it cannot predict rare and catastrophic events and black swans which are not only rare and catastrophic, they are also unimaginable. In the most ironic way possible, this lame excuse is used to justify that sometimes qualitative risk analysis (astrology using heatmaps) is justified. The irony is that qualitative risk analysis techniques have been scientifically proven to be worse than useless.
Let’s translate this excuse into plain English – do not use the techniques which are not perfect some of the time for some of the tasks, instead use the techniques which are guaranteed to be misleading most the time. Solid risk advice if you ask me 🙂
But wait for it, that’s not even the worst part. The argument is actually completely false for the following reasons:
Any risk is a distribution
Any risk on the planet can be represented by a distribution, continuous or discrete, bounded or unbounded, still a distribution. Distribution can represent losses, effects, monetary or non financial, fat tailed or not, still a distribution. This means that any risk may have probability of no impact, some probability that small to moderate effect will happen, some probability large effect will happen and a tiny probability a catastrophic effect will happen.
If you can think of a risk that would not fit any known distribution please write in the comments.
If risk is a distribution, then it will have 3 important components, from left to right:
- expected effect (average)
- unexpected effect (p95 or whatever confidence level is minus average)
- tail (effects beyond confidence level)
Horses for courses, quantification technique should fit the decision
The 3 components of the distribution bring us to an important idea – while risk is a distribution there are multiple ways of estimating the distribution, each with a different accuracy. It could be a basic quantitative risk register (the range of the distribution will be very wide and highly approximate) or it could be a quantitative bow-tie (narrower range, still quite approximate) or a decision tree or a tailor made model (even narrower range, good estimate at the set confidence level). Each applications has own uses. For simplicity sake I call quant risk register – basic, quant bow tie – standardized and tailor made models – advanced.

Horses for courses is a very important principle in risk management. It implies that risk analysis should fit the decision at hand. For example if you need to aggregate ELs for budgeting purposes then a quant risk register is fine. If you need to test different control and mitigation options then have to invest in a bow-tie because risk register is not designed for this. If you need to aggregate ULs as an input into company stress test, then again a risk register will be just fine. If you are trying to save few million on insurance, like we did last year, then have to build a tailor made model, etc, etc.
So if someone ever approached me to quantify rare catastrophic event I probably wouldn’t represent it as a distribution and just quantify the plausible worse case consequence, like we do with explosions for insurance purposes when we use risk engineers who estimate loss scenarios. For everything else, there is Mastercard, I mean quant risk analysis 🙂
Most quant models are designed to work at CI90%, but no more
Different risk quantifications for different decisions and different risk appetites. It is important to bring confidence intervals and confidence levels into the conversation. Most quant models are fine at 90% confidence interval, this means they purposefully exclude 5% best cases and 5% worst cases. This also means that if someone wants to use them for rare and catastrophic event, they will not work.
Let me repeat this very important idea – most models are specifically designed in a way to ignore worst rare catastrophic black swan cases. By design. Because knowing the body of the distribution with even a very high level approximation of the tail can still help decision makers and save a loooooooooooooot of money. We saved $13M just last year with models simpler than I originally anticipated, check out my RAW2022 workshops on this. We actually purposefully went from complex to simplified models. Still saved.
Models at CI90% are used for budgeting, simple mitigations, normal day to day decision making. They ARE NOT used for stress testing, insurance or business continuity.
Does it make risk quantification useless? No silly. It just means models have limitations, which good risk managers are transparent about and disclose them in the methodology section of the risk analysis. And we use models for solving problems they can solve, not the solving every problem under the sun. Taleb said it best, if there is a chance of ruin, better hedge for it.
Did I forget some other excuses or arguments? Write in the comments. Will probably end up adding to this article later, have to run and pick up kids from the school.
Check out other decision making books
RISK-ACADEMY offers online courses
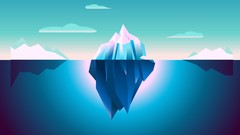
+ Buy now
Informed Risk Taking
Learn 15 practical steps on integrating risk management into decision making, business processes, organizational culture and other activities!
149,9949,99
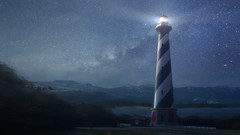
+ Buy now
Управление рисками
В этом коротком и очень увлекательном курсе, Алексей Сидоренко расскажет о причинах внедрения риск менеджмента, об особенностях принятия управленческих решений в ситуации неопределенности и изменениях в новом стандарте ИСО 31000:2018.
49,9919,99