Наши популярные онлайн курсы

A study published in the Annals of Oncology found that a deep learning algorithm achieved a 95% accuracy rate in detecting melanoma from skin lesion images, outperforming a panel of 58 dermatologists whose average accuracy was 86.6%. In another study published in Nature, a deep learning system was able to identify breast cancer from mammograms with greater accuracy than radiologists. The AI system demonstrated a reduction of 1.2% (UK set) and 5.7% (US set) in false positives, and 2.7% (UK set) and 9.4% (US set) in false negatives. Research in the journal JAMA Network Open demonstrated that an AI algorithm was able to diagnose lymph node metastasis in breast cancer patients with an accuracy of 99.5%. This surpassed the 96.9% accuracy demonstrated by human pathologists. Research conducted by Siemens in 2019 demonstrated that AI-driven predictive maintenance tools could forecast equipment failures with up to 30% greater accuracy than experienced maintenance personnel. And according to a 2021 study by J.P. Morgan, AI and machine learning models reduced default prediction errors by approximately 25% over traditional statistical models.
And yet, every time me or one of my team members do a webinar on using AI for risk management, the only question that people ask is “how accurate is AI”. Every bloody time.
So let me share a story. In my last 5 Head of Risk roles, I had access to both world-class team of quant risk professionals and to different AI models, including the in-house built ones. And you know what, I spent considerably more time verifying, checking, correcting and validation my human risk team’s deliverables than I do now verifying RAW@AI deliverables. What used to take my team weeks to do, now can be done by AI + python in hours.
AI doesn’t have to be always right, it just has to be less wrong than humans
In my mind, for AI to be universally adopted, it doesn’t need to be perfect—it just needs to be better than humans at making fewer mistakes. This idea can be linked to the “beat the bear” fallacy described by Douglas Hubbard. Imagine two campers confronted by a bear; one doesn’t have to outrun the bear, just the other camper. Similarly, AI doesn’t have to be flawless; it just needs to outperform human error rates or speed of analysis.
Humans are great at many things, but we can get tired, we can overlook details, we have less exposure to some risks than others and we all have our biases. These limitations make risk managers less effective, especially when dealing with probability theory, complex, interrelated risks and decisions. AI, on the other hand, can handle huge datasets, large volumes of text and complex calculations without getting weary or biased. AI still makes mistakes. That isn’t the question. Less or mistakes than an alternative, that is the right question.
My RAW@AI, for example, can consistently outperform Big 4 risk consultants and most RM1 risk managers. Try it.
The more data you have, the more AI outperforms humans
Large volumes of data is what gives AI its risk management superpower. Unlike humans, AI can quickly go through huge amounts of both structured data (risk registers, spreadsheets and databases) and unstructured data (risk reports, interview transcriptions, annual reports, and research papers). This ability lets AI gather a wide and current view of potential risks. Most risk managers can of course do the same, but it will take them 10X time to achieve comparable level of quality.
The human brain is incredibly adept at recognizing familiar patterns, but it struggles with the sheer complexity and subtlety of patterns found in today’s risk landscapes. AI, especially with deep learning techniques, excels at finding complex and non-linear relationships withing massive datasets. This can reveal hidden connections between seemingly disparate events or data points, highlighting risks that would otherwise go unnoticed until it’s too late. According to a 2022 report by IBM, AI systems detected and responded to security breaches an average of 40% quicker than human-led teams.
You no longer need math PhD to do quant risk analysis
Every time I would join a company I would struggle to find quants who understand risk management and capable of abstract thinking to integrate into decision making. If you ever tried hiring a quant for risk management, you know what I mean.
Well, AI is changing the game. AI models with access to Python environment are putting powerful quantitative tools into the hands of a wider range of professionals. AI models take care of the complex math, allowing risk managers to focus on the insights and implications of the risk analysis rather than the underlying calculations. Just like calculators made complex computations accessible to everyone, AI is doing the same for risk modeling. You don’t need to understand the inner workings of a calculator to get the answer, and you no longer need to be a mathematics whiz to perform sophisticated risk assessments.
You still need to double check the calculations, because calculation errors are frequent. But you know, what is even more frequent? Calculation errors by human risk managers. Much more frequent :)) The question isn’t whether AI will transform risk management. It’s whether you will upskill quickly enough to utilize AI and guide its insights, or your team will be replaced by the next version of RAW@AI.
Learn how to start using AI models in your risk department at #RAW2024.
Important limitations:
- Utilizing AI in risk management involves handling sensitive data, which can raise compliance and privacy issues. Some risks are too sensitive to be analysed by AI, unless it is an in-house closed model.
- Using AI for risk management will probably be considered high risk activity under the EU AI Act and will require significant compliance controls.
- In cases where AI-driven decisions lead to financial losses or compliance breaches, establishing accountability can be challenging. Determining whether the fault lies in the data, model, or decision-making process requires clear protocols.
- Effective use of AI in risk management requires specialized skills that may not be readily available within traditional risk teams. At least hiring or upskilling personnel to work effectively with AI tools is easier than finding a good risk quant who understands decision science and behavioural economics.
RISK-ACADEMY offers online courses
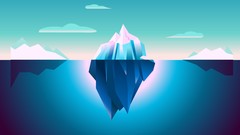
+
Informed Risk Taking
Learn 15 practical steps on integrating risk management into decision making, business processes, organizational culture and other activities!
$149,99$49,99

+
Advanced Risk Governance
This course gives guidance, motivation, critical information, and practical case studies to move beyond traditional risk governance, helping ensure risk management is not a stand-alone process but a change driver for business.
$795